Module 20 Example: Creating a reproducible data pre-processing protocol
We will walk through an example of creating a reproducible data pre-processing protocol. As an example, we will look at how to pre-process and analyze data that are collected in the laboratory to estimate bacterial load in samples. These data come from plating samples from an immunological experiment at serial dilutions, using data from an experiment lead by one of the coauthors. This data pre-processing protocol was created using RMarkdown and allows the efficient, transparent, and reproducible pre-processing of plating data for all experiments in the research group. We will go through how RMarkdown techniques can be applied to develop this type of data pre-processing protocol for a laboratory research group.
Objectives. After this module, you should be able to:
- Explain how a reproducible data pre-processing protocol can be developed for a real research project
- Understand how to design and implement a data pre-processing protocol to replace manual or point-and-click data pre-processing tools
- Apply techniques in RMarkdown to develop your own reproducible data pre-processing protocols
20.1 Introduction and example data
In this module, we’ll provide advice and an example of how you can use the tools for knitted documents to create a reproducible data pre-processing protocol. This module builds on ideas and techniques that were introduced in the last two modules (modules 18 and 19), to help you put them into practical use for data pre-processing that you do repeatedly for research data in your laboratory.
In this module, we will use an example of a common pre-processing task in
immunological research: estimating the bacterial load in samples by plating at
different dilutions. For this type of experiment, the laboratory researcher
plates each of the samples at several dillutions, identifies a good dilution for
counting colony-forming units (CFUs), and then back-calculates the estimated
bacterial load in the original sample based on the colonies counted at this “good”
dilution. This experimental technique dates back to the late 1800s, with Robert
Koch, and continues to be widely used in microbiology research and applications
today.341 These data are originally from an experiment in one
of our authors’ laboratory and are also available as example data for an R
package called bactcountr
, currently under development at
https://github.com/aef1004/bactcountr/tree/master/data.
These data are representative of data often collected in immunological research. For example, you may be testing out some drugs against an infectious bacteria and want to know how successful different drugs are in limiting bacterial load. You run an experiment and have samples from animals treated with different drugs or under control and would then want to know how much viable (i.e., replicating) bacteria are in each of your samples.
You can find out by plating the sample at different dilutions and counting the colony-forming units (CFUs) that are cultured on each plate. You put a sample on a plate with a medium they can grow on and then give them time to grow. The idea is that individual bacteria from the original sample end up randomly around the surface of the plate, and any that are viable (able to reproduce) will form a new colony that, after a while, you’ll be able to see.
To get a good estimate of bactieral load from this process, you need to count CFUs on a “countable” plate—one with a “just right” dilution (and you typically won’t know which dilution this is for a sample until after plating). If you have too high of a dilution (i.e., one with very few viable bacteria), randomness will play a big role in the CFU count, and you’ll estimate the original bacterial load with more variability. If you have too low of a dilution (i.e., one with lots of viable bacteria), it will be difficult to identify separate colonies, and they may complete for resources. To translate from diluted concentration to original concentration, you can then do a back-calculation, incorporating both the number of colonies counted at that dilution and how dilute the sample was. There is therefore some pre-processing required (although it is fairly simple) to prepare the data collected to get an estimate of bacterial load in the original sample. This estimate of bacterial load can then be used in statistical testing and combined with other experimental data to explore questions like whether a candidate vaccine reduces bacterial load when a research animal is challenged with a pathogen.
We will use this example of a common data pre-processing task to show how to create a reproducible pre-processing protocol in this module. If you would like, you can access all the components of the example pre-processing protocol and follow along, re-rendering it yourself on your own computer. The example data are available as a csv file, downloadable here. You can open this file using spreadsheet software, or look at it directly in RStudio. The final pre-processing protocol for these data can also be downloaded, including both the original RMarkdown file and the output PDF document. Throughout this module, we will walk through elements of this document, to provide an example as we explain the process of developing data pre-processing modules for common tasks in your research group. We recommend that you go ahead and read through the output PDF document, to get an idea for the example protocol that we’re creating.
This example is intentionally simple, to allow a basic introduction to the process using pre-processing tasks that are familiar to many laboratory-based scientists and easy to explain to anyone who has not used plating in experimental work. However, the same general process can also be used to create pre-processing protocols for data that are much larger or more complex or for pre-processing pipelines that are much more involved. For example, this process could be used to create data pre-processing protocols for automated gating of flow cytometry data or for pre-processing data collected through single cell RNA sequencing.
20.2 Advice on designing a pre-processing protocol
Before you write your protocol in a knitted document, you should decide on the content to include in the protocol. This section provides tips on this design process. In this section, we’ll describe some key steps in designing a data pre-processing protocol:
- Defining input and output data for the protocol;
- Setting up a project directory for the protocol;
- Outlining key tasks in pre-processing the input data; and
- Adding code for pre-processing.
We will illustrate these design steps using the example protocol on pre-processing plating data.
20.2.1 Defining input and output data for the protocol
The first step in designing the data pre-processing protocol is to decide on the starting point for the protocol (the data input) and the ending point (the data output). It may make sense to design a separate protocol for each major type of data that you collect in your research laboratory. Your input data for the protocol, under this design, might be the data that is output from a specific type of equipment (e.g., flow cytometer) or from a certain type of sample or measurement (e.g., metabolomics run on a mass spectrometer), even if it is a fairly simple type of data (e.g., CFUs from plating data, as used in the example protocol for this module). For example, say you are working with three types of data for a research experiment: data from a flow cytometer, metabolomics data measured with a mass spectrometer, and bacterial load data measured by plating data and counting colony forming units (CFUs). In this case, you may want to create three pre-processing protocols: one for the flow data, one for the metabolomics data, and one for the CFU data. These protocols are modular and can be re-used with other experiments that use any of these three types of data.
With an example dataset, you can begin to create a pre-processing protocol before you collect any of your own research data for a new experiment. If the format of the initial data is similar to the format you anticipate for your data, you can create the code and explanations for key steps in your pre-processing for that type of data. Often, you will be able to adapt the RMarkdown document to change it from inputting the example data to inputting your own experimental data with minimal complications, once your data comes in. By thinking through and researching data pre-processing options before the data is collected, you can save time in analyzing and presenting your project results once you’ve completed the experimental data collection for the project. Further, with an example dataset, you can get a good approximation of the format in which you will output data from the pre-processing steps. This will allow you to begin planning the analysis and visualization that you will use to combine the different types of data from your experiment and use it to investigate important research hypotheses. Again, if data follow standardized formats across steps in your process, it will often be easy to adapt the code in the protocol to input the new dataset that you created, without major changes to the code developed with the example dataset.
While pre-processing protocols for some types of data might be very complex, others might be fairly simple. However, it is still worthwhile to develop a protocol even for simple pre-processing tasks, as it allows you to pass along some of the details of pre-processing the data that might have become “common sense” to longer-tenured members of your research group. For example, the pre-processing tasks in the example protocol are fairly simple. This protocol inputs data collected in a plain-text delimited file (a csv file, in the example). Within the protocol, there are steps to convert initial measurements from plating at different dilutions into an estimate of the bacterial load in each sample. There are also sections in the protocol for exploratory data analysis, to allow for quality assessment and control of the collected data as part of the pre-processing. The output of the protocol is a simple data object (a dataframe, in this example) with the bacterial load for each original sample. These data are now ready to be used in tables and figures in the research report or manuscript, as well as to explore associations with the experimental design details (e.g., comparing bacterial load in treated versus untreated animals) or merged with other types of experimental data (e.g., comparing immune cell populations, as measured with flow cytometry data, with bacterial loads, as measured from plating and counting CFUs).
Once you have identified the input data type to use for the protocol, you should identify an example dataset from your laboratory that you can use to create the protocol. This could be a dataset that you currently need to pre-process, in which case the development of the protocol will serve a second purpose, allowing you to complete this task at the same time. However, you may not have a new set of data of this type that you currently need to pre-process, and in this case you can build your protocol using a dataset from a previous experiment in your laboratory. In this case, you may already have a record of the steps that you used to pre-process the data previously, and these can be helpful as a starting point as you draft the more thorough pre-processing protocol. You may want to select an example dataset that you have already published or are getting ready to publish, so you won’t feel awkard about making the data available for people to practice with. If you don’t have an example dataset from your own laboratory, you can explore example datasets that are already available, either as data included with existing R packages or through open repositories, including those hosted through national research institutions like the NIH. In this case, be sure to cite the source of the data and include any available information about the equipment that was used to collect it, including equipment settings used when the data were collected.
For the example protocol for this module, we want to pre-process data that were collected “by hand” by counting CFUs on plates in the laboratory. These counts were recorded in a plain text delimited file (a csv file) using spreadsheet software. The spreadsheet was set up to ensure the data can easily be converted to a “tidy” format, as described in module 3. The first few rows of the input data look like this:
## # A tibble: 6 × 6
## group replicate dilution_0 dilution_1 dilution_2 dilution_3
## <dbl> <chr> <chr> <chr> <dbl> <dbl>
## 1 2 2-A 26 10 0 0
## 2 2 2-B TNTC 52 10 5
## 3 2 2-C 0 0 0 0
## 4 3 3-A 0 0 0 0
## 5 3 3-B TNTC TNTC 30 10
## 6 3 3-C 0 0 0 0
Each row represents the number of bacterial colonies counted after plating a
certain sample, where each sample represents one experimental animal and several
experimental animals (replicates) were considered for each experimental group.
Columns are included with values for the experimental group of the sample
(group
), the specific ID of the sample within that experimental group
(replicate
, e.g., 2-A
is mouse A in experimental group 2), and the
colony-forming units (CFUs) counted at each of several dilutions. If a cell has
the value “TNTC”, this indicates that CFUs were too numerous to count for that
sample at that dilution.
When you have identified the input data type you will use for the protocol, as well as selected an example dataset of this type to use to create the protocol, you can include a section in the protocol that describes these input data, what file format they are in, and how they can be read into R for pre-processing (Figure 20.1).
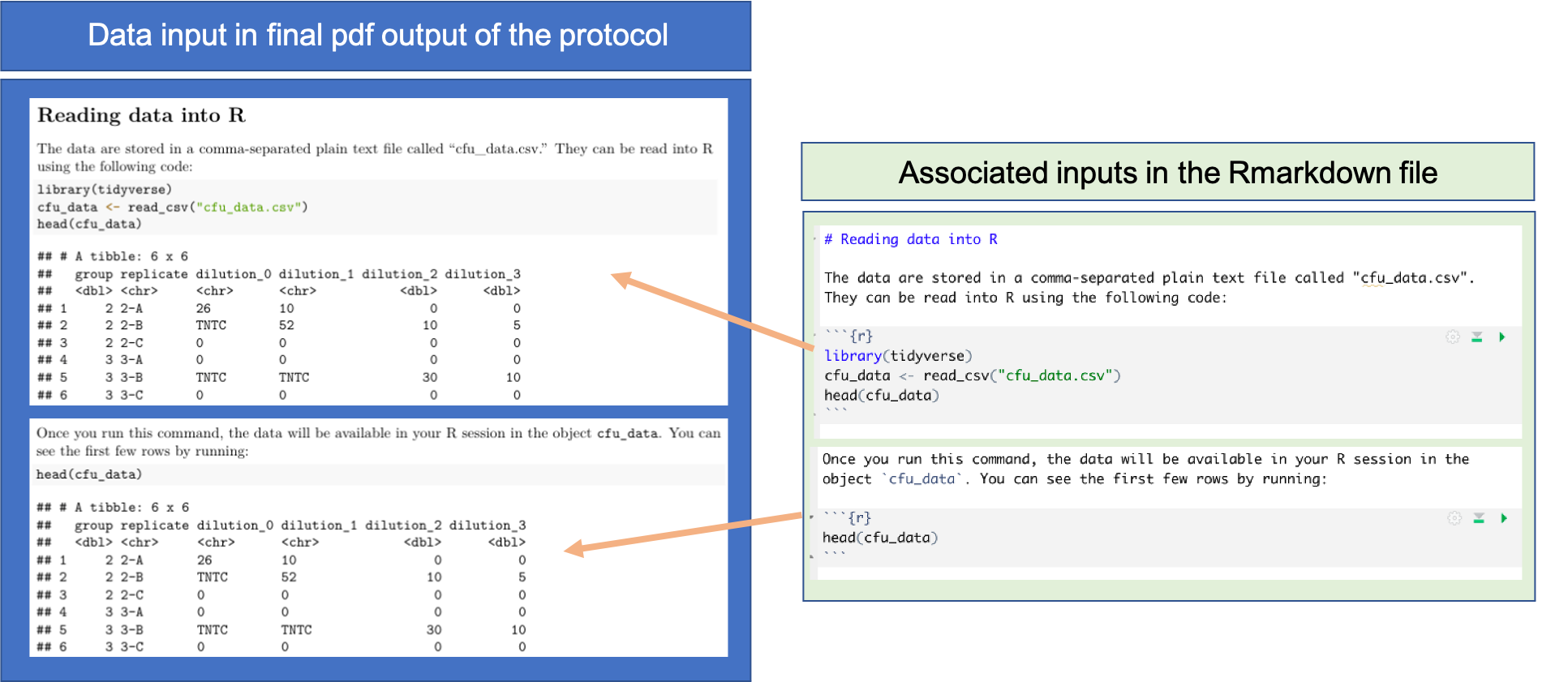
Figure 20.1: Providing details on input data in the pre-processing protocol. Once you have an example data file for the type of data that will be input for the protocol, you can add a section that provides the code to read the data into R. You can also add code that will show the first few rows of the example dataset, as well as a description of the data. This figure shows examples of how these elements can be added to an RMarkdown file for a pre-processing protocol, and the associated elements in the final pdf of the protocol, using the example protocol for this module.
For the data output, it often makes sense to plan for data in a format that is appropriate for data analysis and for merging with other types of data collected from the experiment. The aim of pre-processing is to get the data from the format in which they were collected into a format that is meaningful for combining with other types of data from the experiment and using in statistical hypothesis testing.
In the example pre-processing protocol, we ultimately output a simple dataset, with one row for each of the original samples. The first few rows of this output data are:
## # A tibble: 6 × 3
## group replicate cfu_in_organ
## <dbl> <chr> <dbl>
## 1 2 2-A 260
## 2 2 2-B 2500
## 3 2 2-C 0
## 4 3 3-A 0
## 5 3 3-B 7500
## 6 3 3-C 0
For each original sample, an estimate of the CFUs of Mycobacterium
tuberculosis in the full spleen is given (cfu_in_organ
). These data
can now be merged with other data collected about each animal in the experiment.
For example, they could be joined with data that provide measures of the
immune cell populations for each animal, to explore if certain immune
cells are associated with bacterial load. They could also be joined with
experimental information and then used in hypothesis testing. For example,
these data could be merged with a table that describes which groups were
controls versus which used a certain vaccine, and then a test could be
conducted exploring evidence that bacterial loads in animals given a
vaccine were lower than in control animals.
20.2.2 Setting up a project directory for the protocol
Once you have decided on the input and output data formats, you will next want to set up a file directory for storing all the inputs needed in the protocol. You can include the project files for the protocol in an RStudio Project (see module 6) and post this either publicly or privately on GitHub (see modules 9–11). This creates a “packet” of everything that a reader needs to use to recreate what you did—they can download the whole GitHub repository and will have a nice project directory on their computer with everything they need to try out the protocol.
Part of the design of the protocol involves deciding on the files that should be included in this project directory. Figure 20.2 provides an example of the initial files included in the project directory for the example protocol for this module. The left side of the figure shows the files that are initially included, while the left side shows the files in the project after the code in the protocol is run.
Generally, in the project directory you should include a file with the input example data, in whatever file format you will usually collect this type of data. You will also include an RMarkdown file where the protocol is written. If you are planning to cite articles and other references, you can include a BibTeX file, with the bibliographical information for each source you plan to cite (see module 19). Finally, if you would like to include photographs or graphics, you can include these image files in the project directory. Often, you might want to group these together in a subdirectory of the project named something like “figures”.
Once you run the RMarkdown file for the protocol, you will generate additional files in the project. Two typical files you will generate will be the output file for the protocol (in the example, this is output to a pdf file). Usually, the code in the protocol will also result in output data, which is pre-processed through the protocol code and written into a file to be used in further analysis.
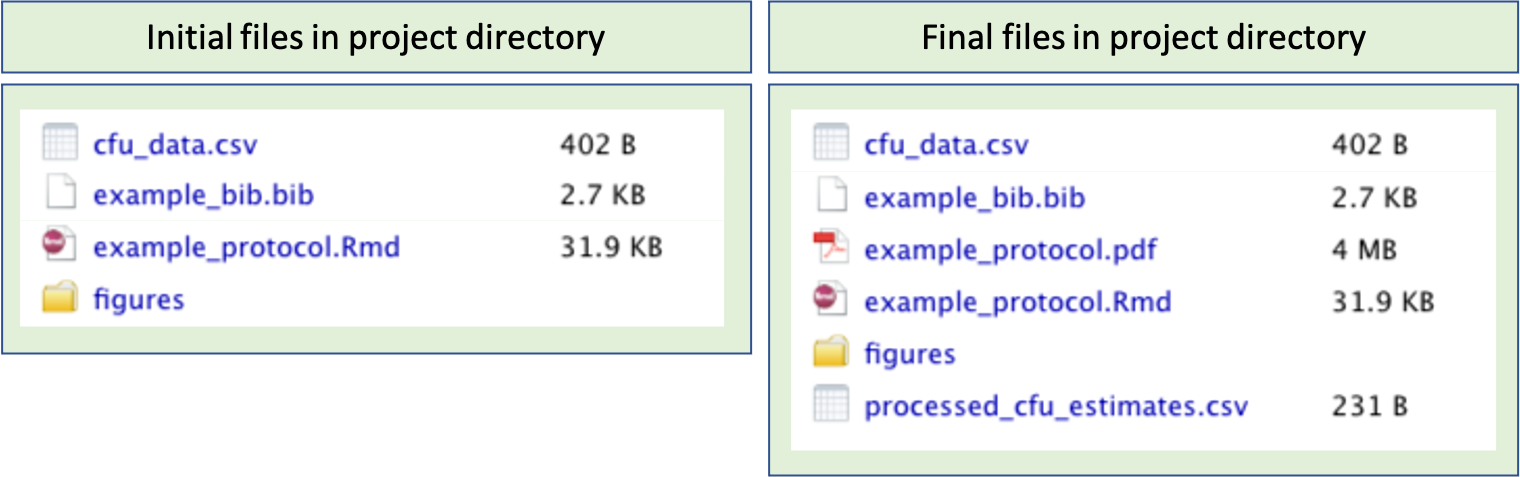
Figure 20.2: Example of files in the project directory for a data pre-processing protocol. On the left are the files initially included in the project directory for the example protocol for this module. These include a file with the input data (cfu_data.csv), a BibTeX file with bibliographical information for references (example_bib.bib), the RMarkdown file for the protocol (example_protocol.Rmd), and a subdirectory with figures to include in the protocol (figures). On the right is shown the directory after the code in the protocol RMarkdown document is run, which creates an output pdf with the protocol (example_protocol.pdf) as well as the output data (processed_cfu_estimates.csv).
20.2.3 Outlining key tasks in pre-processing the input data
The next step is to outline the key tasks that are involved in moving from the data input to the desired data output. For the plating data we are using for our example, the key tasks to be included in the pre-processing protocol are:
- Read the data into R
- Explore the data and perform some quality checks
- Identify a “good” dilution for each sample—one at which you have a countable plate
- Estimate the bacterial load in each original sample based on the CFUs counted at that dilution
- Output data with the estimated bacterial load for each sample
Once you have this basic design, you can set up the RMarkdown file for the
pre-processing protocol to include a separate section for each task, as well as
an “Overview” section at the beginning to describe the overall protocol, the
data being pre-processed, and the laboratory procedures used to collect those
data. In RMarkdown, you can create first-level section headers by putting the
text for the header on its own line and beginning that line with #
, followed
by a space. You should include a blank line before and after the line with this
header text. Figure 20.3 shows how this is done in the
example protocol for this module, showing how text in the plain text RMarkdown
file for the protocol align with section headers in the final pdf output of the
protocol.
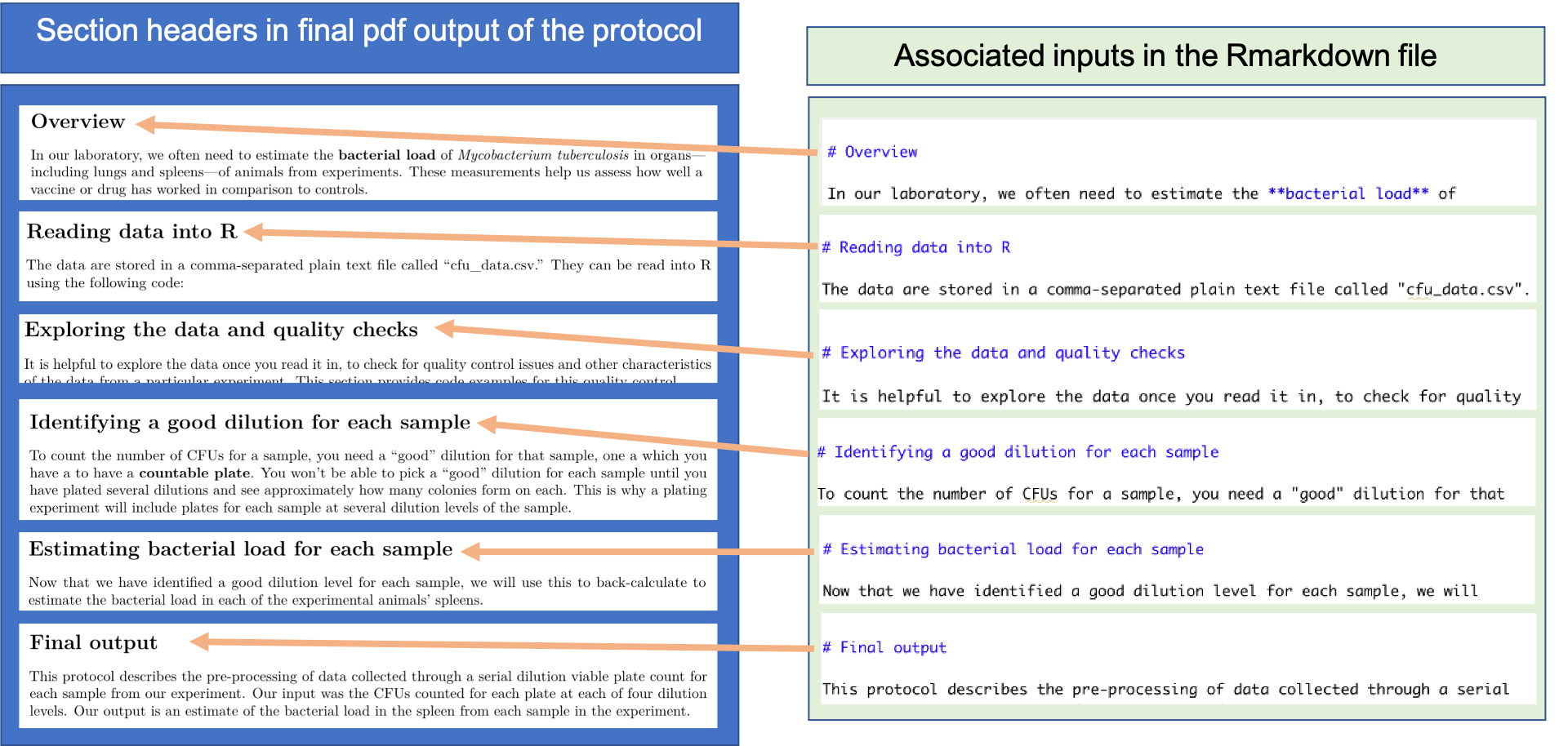
Figure 20.3: Dividing an RMarkdown data pre-processing protocol into sections. This shows an example of creating section headers in a data pre-processing protocol created with RMarkdown, showing section headers in the example pre-procotcol for this module.
20.2.4 Adding code for pre-processing
For many of these steps, you likely have code—or can start drafting the code—required for that step. In RMarkdown, you can test this code as you write it. You insert each piece of executable code within a special section, separated from the regular text with special characters, as described in previous modules.
For any pre-processing steps that are straightforward (e.g., calculating the dilution factor in the example module, which requires only simple mathematical operations), you can directly write in the code required for the step. For other pre-processing steps, however, the algorithm may be a bit more complex. For example, complex algorithms have been developed for steps like peak identification and alignment that are required when pre-processing data from a mass spectrometer.
For these more complex tasks, you can start to explore available R packages for performing the task. There are thousands of packages available that extend the basic functionality of R, providing code implementations of algorithms in a variety of scientific fields. Many of the R packages relevant for biological data—especially high-throughput biological data—are available through a repository called Bioconductor. These packages are all open-source (so you can explore their code if you want to) and free. You can use vignettes and package manuals for Bioconductor packages to identify the different functions you can use for your pre-processing steps. Once you have identified a function for the task, you can use the helpfile for the function to see how to use it. This help documentation will allow you to determine all of the function’s parameters and the choices you can select for each.
You can add each piece of code in the RMarkdown version of the protocol using the standard method for RMarkdown (module 11). Figure 20.4 shows an example from the example protocol for this module. Here, we are using code to help identify a “good” dilution for counting CFUs for each sample. The code in included in an executable code chunk, and so it will be run each time the protocol is rendered. Code comments are included in the code to provide finer-level details about what the code is doing.
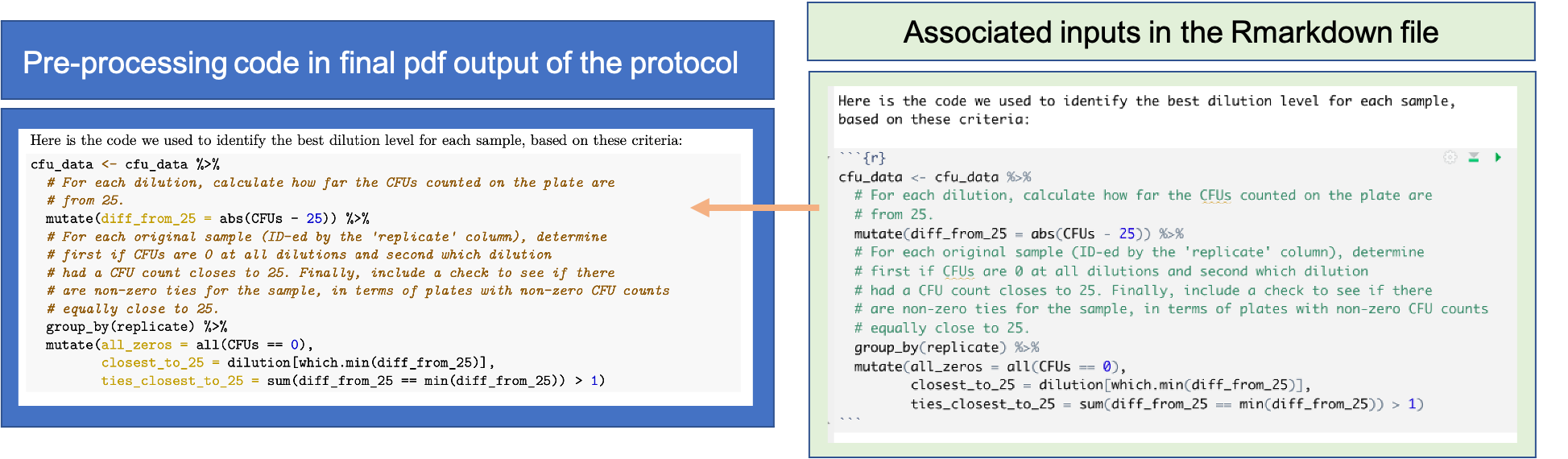
Figure 20.4: Example of including code in a data pre-processing protocol created with RMarkdown. This figure shows how code can be included in the RMarkdown file for a pre-processing protocol (right), and the corresponding output in the final pdf of the protocol (left), for the code to identify a ‘good’ dilution for counting CFUs for each sample. Code comments are included to provide finer-level details on the code.
For each step of the protocol, you can also include potential problems that might come up in specific instances of the data you get from future experiments. This can help you adapt the code in the protocol in thoughtful ways as you apply it in the future to new data collected for new studies and projects.
20.3 Writing data pre-processing protocols
Now that you have planned out the key components of the pre-processing protocol, you can use RMarkdown’s functionality to flesh it out into a full pre-processing protocol. This gives you the chance to move beyond a simple code script, and instead include more thorough descriptions of what you’re doing at each step and why you’re doing it. You can also include discussions of potential limitations of the approach that you are taking in the pre-processing, as well as areas where other research groups might use a different approach. These details can help when it is time to write the Methods section for the paper describing your results from an experiment using these data. They can also help your research group identify pre-processing choices that might differ from other research groups, which opens the opportunity to perform sensitivity analyses regarding these pre-processing choices and ensure that your final conclusions are robust across multiple reasonable pre-processing approaches.
Protocols are common for wet lab techniques, where they provide a “recipe” that ensures consistency and reproducibility in those processes. Computational tasks, including data pre-processing, can also be standardized through the creation and use of protocol in your research group. While code scripts are becoming more common as a means of recording data pre-processing steps, they are often not as clear as a traditional protocol, in particular in terms of providing a thorough description of what is being done at each step and why it is being done that way. Data pre-processing protocols can provide these more thorough descriptions, and by creating them with RMarkdown or with similar types of “knitted” documents (modules 18 and 19), you can combine the executable code used to pre-process the data with extensive documentation. As a further advantage, the creation of these protocols will ensure that your research group has thought carefully about each step of the process, rather than relying on cobbling together bits and pieces of code they’ve found but don’t fully understand. Just as the creation of a research protocol for a clinical trial requires a careful consideration of each step of the ultimate trial,342 the creation of data pre-processing protocols ensure that each step in the process is carefully considered, and so helps to ensure that each step of this process is conducted as carefully as the steps taken in designing the experiment as a whole and each wet lab technique conducted for the experiment.
A data pre-processing protocol, in the sense we use it here, is essentially an annotated recipe for each step in preparing your data from the initial, “raw” state that is output from the laboratory equipment (or collected by hand) to a state that is useful for answering important research questions. The exact implementation of each step is given in code that can be re-used and adapted with new data of a similar format. However, the code script is often not enough to helpfully understand, share, and collaborate on the process. Instead, it’s critical to also include descriptions written by humans and for humans. These annotations can include descriptions of the code and how certain parameters are standardized the algorithms in the code. They can also be used to justify choices, and link them up both with characteristics of the data and equipment for your experiment as well as with scientific principles that underlie the choices. Protocols like this are critical to allow you to standardize the process you use across many samples from one experiment, across different experiments and projects in your research laboratory, and even across different research laboratories.
As you begin adding text to your pre-processing protocol, you should keep in mind these general aims. First, a good protocol provides adequate detail that another researcher can fully reproduce the procedure.343 For a protocol for a trial or wet lab technique, this means that the protocol should allow another researcher to reproduce the process and get results that are comparable to your results;344 for a data pre-processing protocol, the protocol must include adequate details that another researcher, provided they start with the same data, gets identical results (short of any pre-processing steps that include some element of sampling or random-number generation, e.g., Monte Carlo methods). This idea—being able to exactly re-create the computational results from an earlier project—is referred to as computational reproducibility and is considered a key component in ensuring that research is fully reproducible.
By creating the data pre-processing protocol as a knitted document using a tool like RMarkdown (modules 18 and 19), you can ensure that the protocol is computationally reproducible. In an RMarkdown document, you include the code examples as executable code—this means that the code is run every time you render the document. You are therefore “checking” your code every time that you run it. As the last step of your pre-processing protocol, you should output the copy of the pre-processed data that you will use for any further analysis for the project. You can use functions in R to output this to a plain text format, for example a comma-separated delimited file (modules 4 and 5). Each time you render the protocol, you will re-write this output file, and so this provides assurance that the code in your protocol can be used to reproduce your output data (since that’s how you yourself created that form of the data).
Figure 20.5 provides an example from the example protocol for this module. The RMarkdown file for the protocol includes code to write out the final, pre-processed data to a comma-separated plain text file called “processed_cfu_estimates.csv”. This code writes the output file into the same directory where you’ve saved the RMarkdown file. Each time the RMarkdown file is rendered to create the pdf version of the protocol, the input data will be pre-processed from scratch, using the code throughout the protocol, and this file will be overwritten with the data generated. This guarantees that the code in the protocol can be used by anyone—you or other researchers—to reproduce the final data from the protocol, and so guarantees that these data are computationally reproducible.
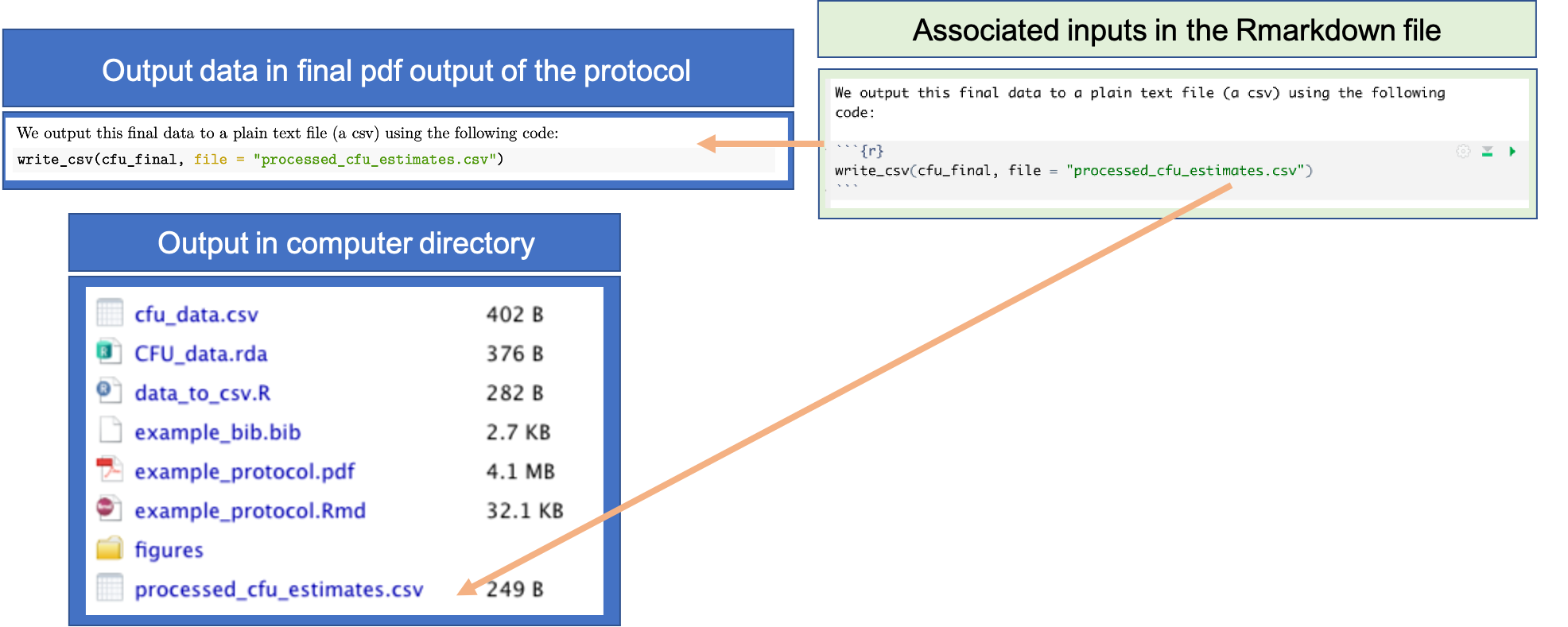
Figure 20.5: Example of using code in pre-processing protocol to output the final, pre-processed data that will be used in further analysis for the research project. This example comes from the example protocol for this module, showing both the executable code included in the RMarkdown file for the protocol (right) and how this code is included in the final pdf of the protocol. Outputting the pre-processed data into a plain text file as the last step of the protocol helps ensure computational reproducibility for this step of working with experimental data.
In your data pre-processing protocol, show the code that you use to implement this choice and also explain clearly in the text why you made this choice and what alternatives should be considered if data characteristics are different. Write this as if you are explaining to a new research group member (or your future self) how to think about this step in the pre-processing, why you’re doing it the way your doing it, and what code is used to do it that way. You should also include references that justify choices when they are available—include these using BibTeX (module 19). By doing this, you will make it much easier on yourself when you write the Methods section of papers that report on the data you have pre-processed, as you’ll already have draft information on your pre-processing methods in your protocol.
Good protocols include not only how (for data pre-processing protocols, this is the code), but also why each step is taken. This includes explanations that are both higher-level (i.e., why a larger question is being asked) and also at a fine level, for each step in the process. A protocol should include some background, the aims of the work, hypotheses to be tested, materials and methods, methods of data collection and equipment to analyze samples.345
This step of documentation and explanation is very important to creating a useful data pre-processing protocol. Yes, the code itself allows someone else to replicate what you did. However, only those who are very, very familiar with the software program, including any of the extension packages you include, can “read” the code directly to understand what it’s doing. Further, even if you understand the code very well when you create it, it is unlikely that you will stay at that same level of comprehension in the future, as other tasks and challenges take over that brain space. Explaining for humans, in text that augments and accompanies the code, is also important because function names and parameter names in code often are not easy to decipher. While excellent programmers can sometimes create functions with clear and transparent names, easy to translate to determine the task each is doing, this is difficult in software development and is rare in practice. Human annotations, written by and for humans, are critical to ensure that the steps will be clear to you and others in the future when you revisit what was done with this data and what you plan to do with future data.
The process of writing a protocol in this way forces you to think about each step in the process, why you do it a certain way (include parameters you choose for certain functions in a pipeline of code), and include justifications from the literature for this reasoning. If done well, it should allow you to quickly and thoroughly write the associated sections of Methods in research reports and manuscripts and help you answer questions and challenges from reviewers. Writing the protocol will also help you identify steps for which you are uncertain how to proceed and what choices to make in customizing an analysis for your research data. These are areas where you can search more deeply in the literature to understand implications of certain choices and, if needed, contact the researchers who developed and maintained associated software packages to get advice.
For example, the example protocol for this module explains how to pre-process data collected from counting CFUs after plating serial dilutions of samples. One of the steps of pre-processing is to identify a dilution for each sample at which you have a “countable” plate. The protocol includes an explanation of why it is important to identify the dilution for a countable plate and also gives the rules that are used to pick a dilution for each sample, before including the code that implements those rules. This allows the protocol to provide research group members with the logic behind the pre-processing, so that they can adapt if needed in future experiments. For example, the count range of CFUs used for the protocol to find a good dilution is about a quarter of the typically suggested range for this process, and this is because this experiment plated each sample on a quarter of a plate, rather than using the full plate. By explaining this reasoning, in the future the protocol could be adapted when using a full plate rather than a quarter of a plate for each sample.
One tool in RMarkdown that is helpful for this process is its built-in referencing system. In the previous module, we showed how you can include bibliographical references in an RMarkdown file. When you write a protocol within RMarkdown, you can include references in this way to provide background and support as you explain why you are conducting each step of the pre-processing. Figure 20.6 shows an example of the elements you use to do this, showing each element in the example protocol for this module.
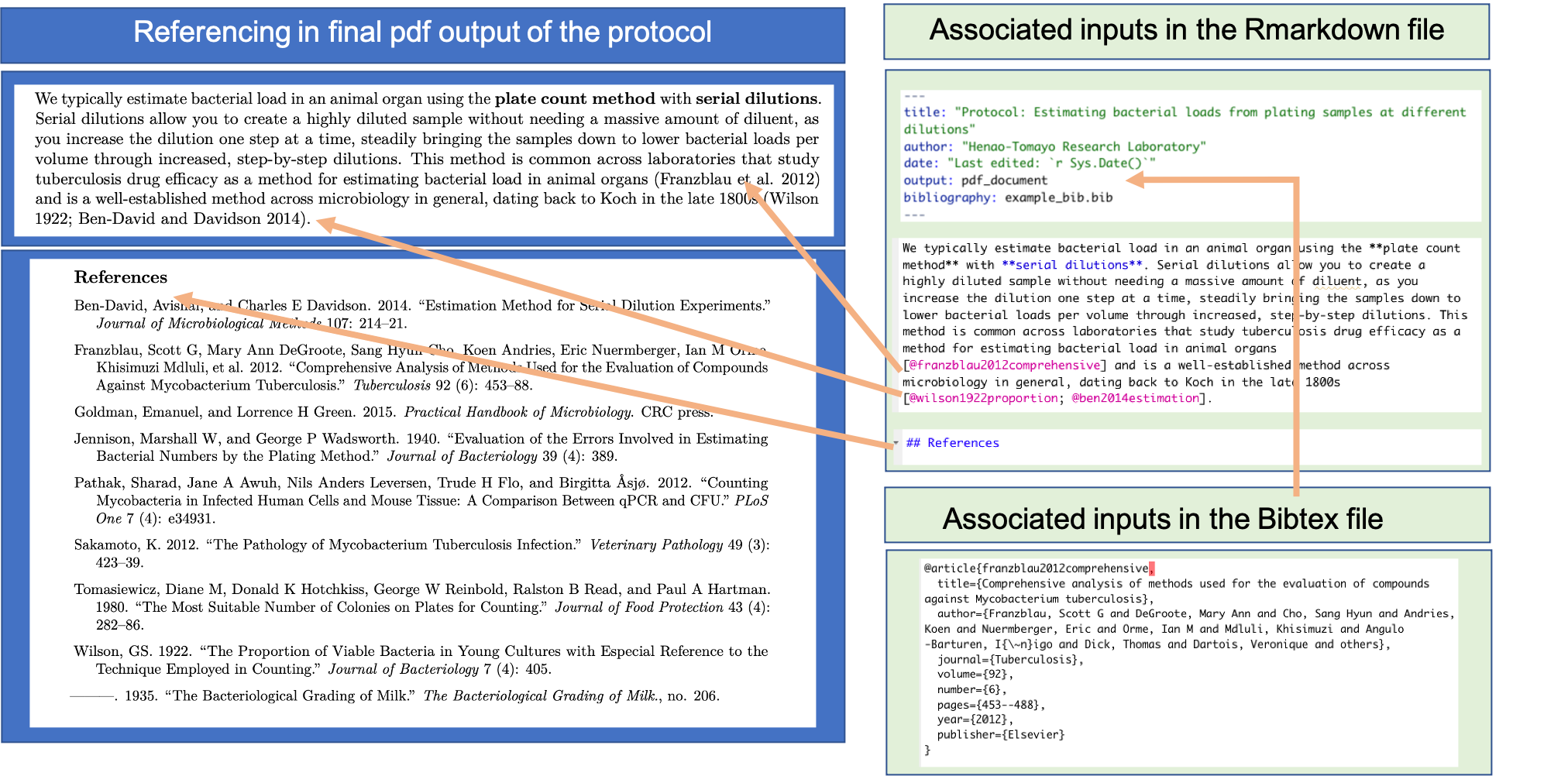
Figure 20.6: Including references in a data pre-processing protocol created with RMarkdown. RMarkdown has a built-in referencing system that you can use, based on the BibTeX system for LaTeX. This figure shows examples from the example protocol for this module of the elements used for referencing. You create a BibTeX file with information about each reference, and then use the key for the reference within the text to cite that reference. All cited references will be printed at the end of the document; you can chose the header that you want for this reference section in the RMarkdown file (‘References’ in this example). In the YAML of the RMarkdown file, you specify the path to the BibTeX file (with the ‘bibliography:’ key), so it can be linked in when the RMarkdown file is rendered.
Other helpful tools in RMarkdown are tools for creating equations and tables. As
described in module 19, RMarkdown includes a number of formatting
tools. You can create simple tables through basic formatting, or more complex
tables using add-on packages like kableExtra
. Math can be typeset using
conventions developed in the LaTeX mark-up language. The module 19
provided advice and links to resources on using these types of tools. Figure
20.7 gives an example of them in use within the example
protocol for this module.
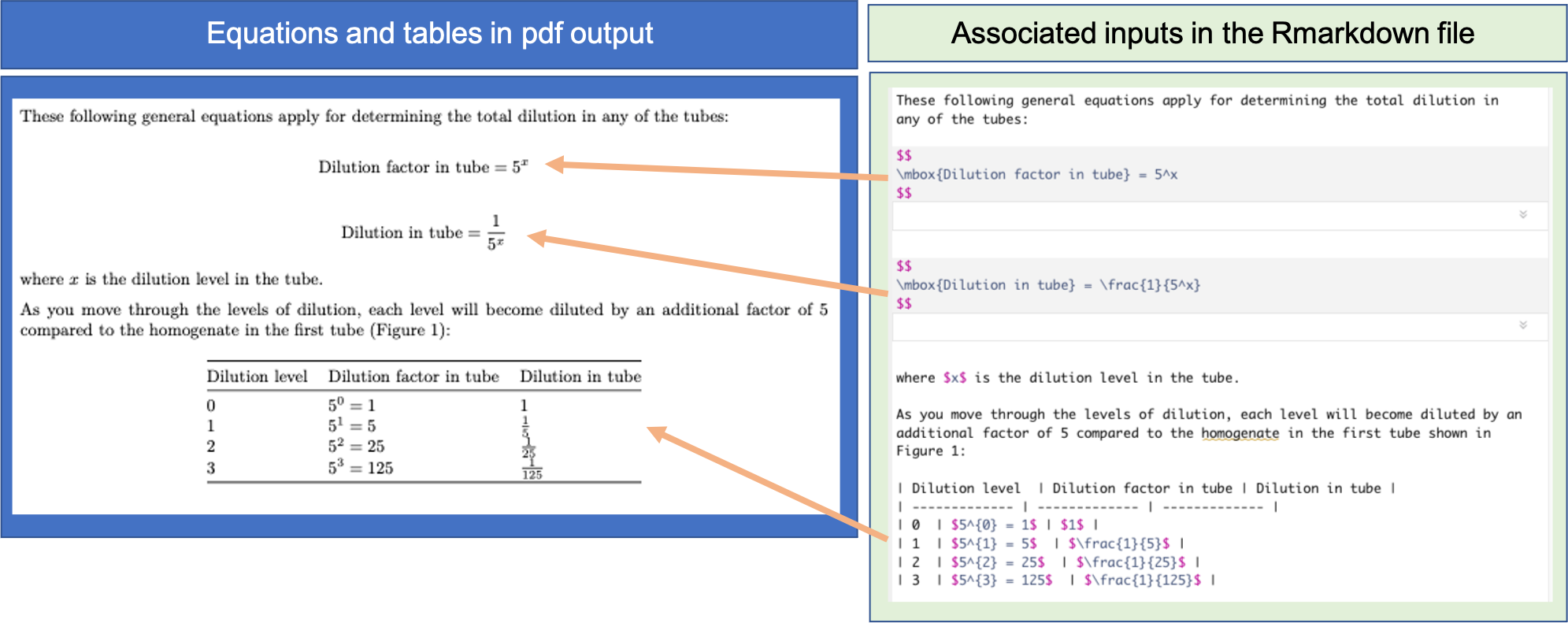
Figure 20.7: Example of including tables and equations in an RMarkdown data pre-processing protocol.
You can also include figures, either figures created in R or outside figure files.
Any figures that are created by code in the RMarkdown document will automatically
be included in the protocol. For other graphics, you can include image files
(e.g., png and jpeg files) using the include_graphics
function from the
knitr
package. You can use options in the code chunk options to specify
the size of the figure in the document and to include a figure caption. The
figures will be automatically numbered in the order they appear in the protocol.
Figure 20.8 shows an example of how external figure files were included in the example protocol. In this case, the functionality allowed us to include an overview graphic that we created in PowerPoint and saved as an image as well as a photograph taken by a member of our research group.
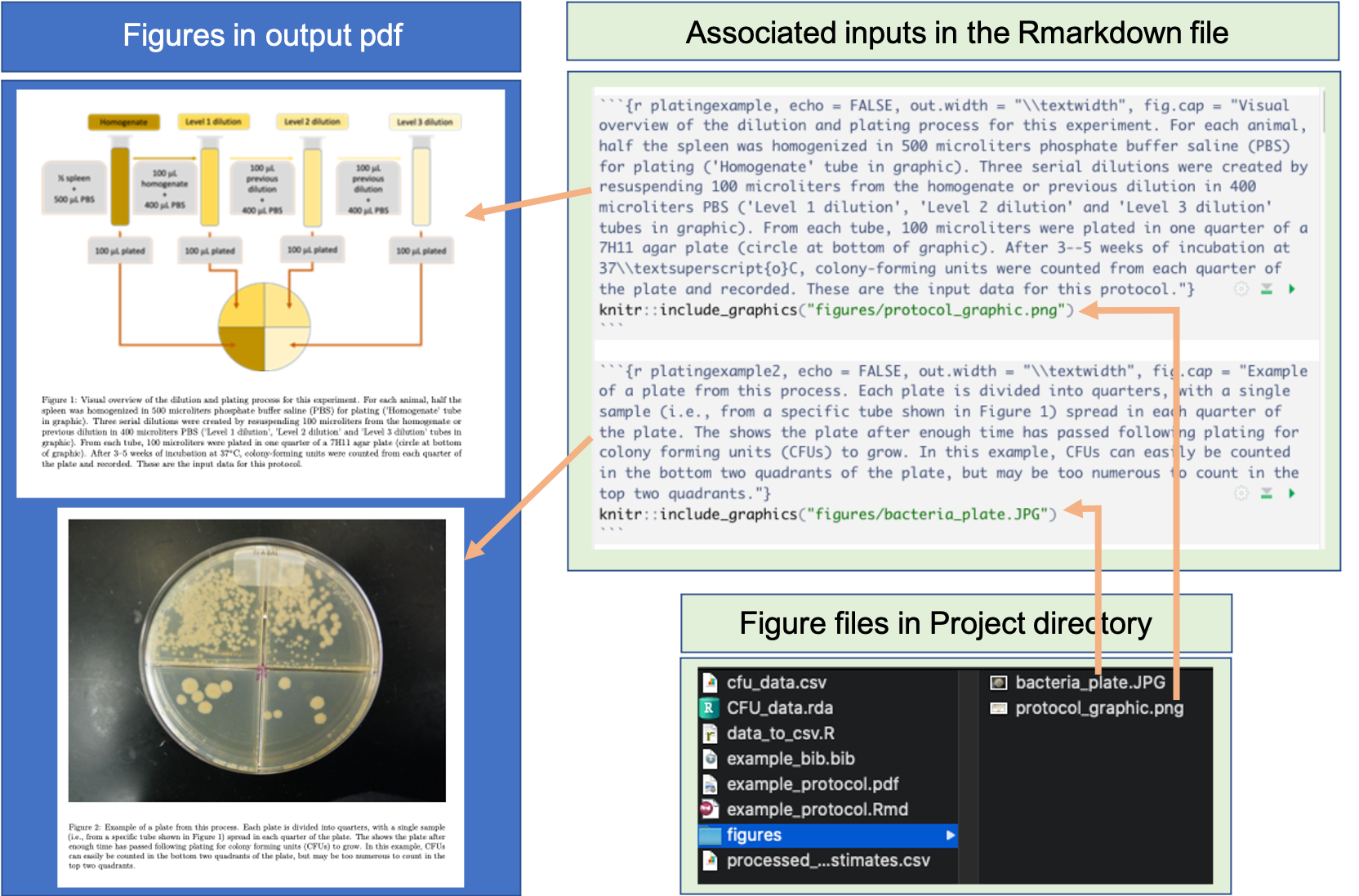
Figure 20.8: Example of including figures from image files in an RMarkdown data pre-processing protocol.
Finally, you can try out even more complex functionality for RMarkdown as you continue to build data pre-processing protocols for your research group. Figure 20.9 shows an example of using R code within the YAML of the example protocol for this module; this allows us to include a “Last edited” date that is updated with the day’s date each time the protocol is re-rendered.

Figure 20.9: Example of using more advanced RMarkdown functionality within a data pre-processing protocol. In this example, R code is incorporated into the YAML of the document to include the date that the document was last rendered, marking this on the pdf output as the Last edited date of the protocol.
20.4 Applied exercise
To wrap up this module, try downloading both the source file and the output of this example data pre-processing protocol. Again, you can find the source code (the RMarkdown file) here and the output file here. If you would like to try re-running the file, you can get all the additional files you’ll need (the original data file, figure files, etc.) here. See if you can compare the elements of the RMarkdown file with the output they produce in the PDF file. Read through the descriptions of the protocol. Do you think that you could recreate the process if your laboratory ran a new experiment that involved plating samples to estimate bacterial load?